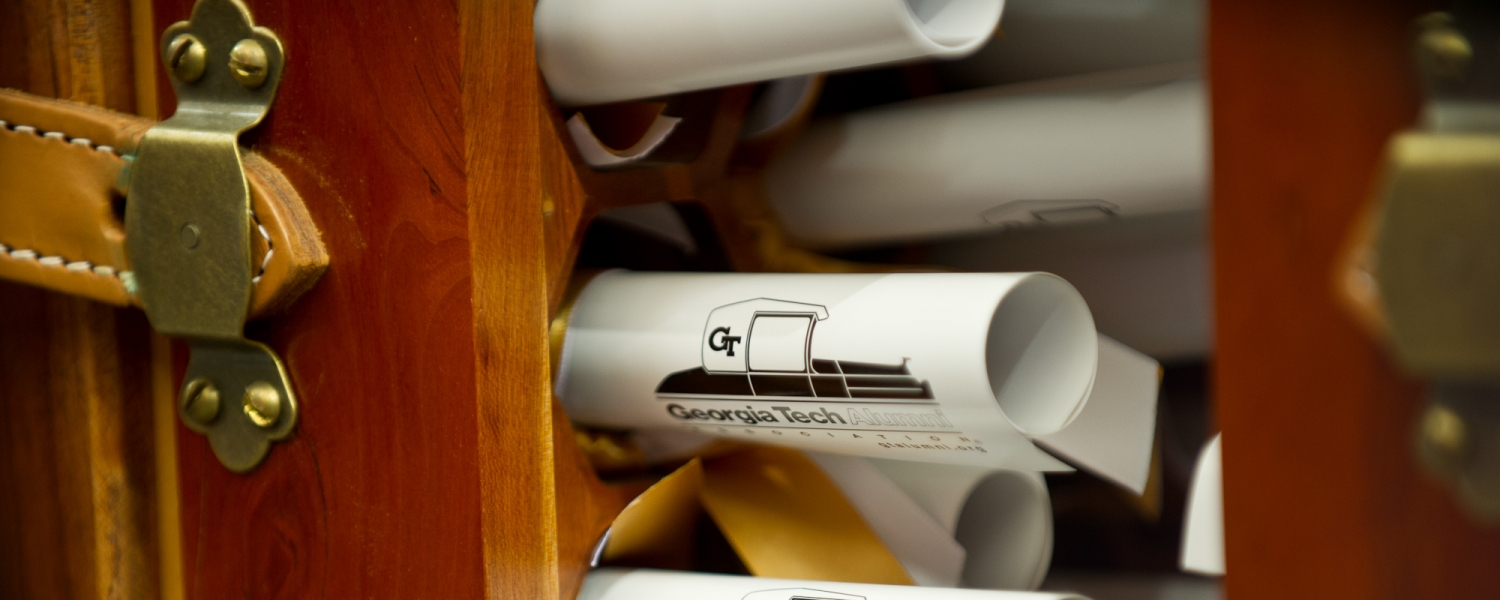
When single-celled bacteria exist in communities known as biofilms, they develop the ability to exhibit complex emergent properties such as social cooperation, resource capture, and enhanced survivability, the individual limitations of existence can be overcome which would otherwise be unlikely. Properties such as matrix production, quorum sensing, and coordinated lifecycle offers structural and functional advantages which makes them highly successful at evading destruction by antimicrobials and immune defenses. With few, if any, novel antibiotics in the clinical pipeline, there is a resurgence of interest in alternatives such as phage therapy, the practice of bacterial viruses known as bacteriophages that infect and lyse bacteria to treat infections.
In this thesis, we explore the understudied impact of phage titer on biofilm dynamics and outcomes. We determined that the biofilm developmental stage at the time of phage addition modulates its response. These responses vary as a function of the phage dose and can be broadly organized into four distinct classes. While nearly any phage dosage is sufficient to suppress biofilm formation in the first developmental stage, very little impact on biofilm outcome is evident for the dispersion stage even at high phage exposures. We also found that the early biofilm stage is increasingly suppressed by higher phage exposures, while paradoxically, the mature biofilm stage is enhanced by increasing phage exposure. Despite this apparently undesired outcome, the inhibition of biofilm dispersion in phage-treated samples could potentially minimize the further spread of infections to other locations. Additionally, for each of the response classes, high phage exposure halts the biofilm from transitioning into the next developmental stage of the biofilm lifecycle. Our results comprehensively demonstrate predictable biofilm outcomes versus phage dosage and biofilm age. These insights combined with the measurements of the rich spatiotemporal dynamics of the biofilms will facilitate future mathematical modeling and experimental efforts to test mechanistic hypotheses, while at the same time provide more perspective to guide clinical studies and phage-based personalized treatments. Other parts of this thesis furthers understanding and approaches to study neutrophil-biofilm interactions, phage lysis in spatially constrained environments, and anti-biofilm properties of a biocompatible hyaluronic acid based polymer brush system. Collectively, this dissertation provides insights on the advantages and limitations of phage, immune cells, and polymer brushes to inhibit, control, and eliminate biofilms.
Event Details
Date/Time:
Characterizing how viruses interact with microbial hosts is critical to understanding microbial community structure and function. However, existing methods for quantifying bacteria-phage interactions are not widely applicable to natural communities. First, many bacteria are not culturable, preventing direct experimental testing. Second, “-omics” based methods, while high in accuracy and specificity, have been shown to be extremely low in power. Third, inference methods based on time-series or co-occurrence data, while promising, have for the most part not been rigorously tested. This thesis work focuses on this final category of quantification strategies: inference methods.
In this thesis, we further our understanding of both the potential and limitations of several inference methods, focusing primarily on time-series data with high time resolution. We emphasize the quantification of efficacy by using time-series data from multi-strain bacteria-phage communities with known infection networks. We employ both in silico simulated bacteria-phage communities as well as an in vitro community experiment. We review existing correlation-based inference methods, extend theory and characterize tradeoffs for model-based inference which uses convex optimization, characterize pairwise interactions in a 5x5 virus-microbe community experiment using Markov chain Monte Carlo, and present analytic tools for microbiome time-series analysis when a dynamical model is unknown. Together, these chapters bridge gaps in existing literature in inference of ecological interactions from time-series data.
Event Details
Date/Time:
Abstract
We often find ourselves working with systems for which governing equations are unknown, or if they are known, they may be high-dimensional to the point of being difficult to analyze and prohibitively expensive to make predictions with. These difficulties, together with the ever-increasing availability of data, have led to the new paradigm of data-driven model discovery. I will present recent work that fruitfully combines a classical idea from applied mathematics with modern methods of machine learning to learn minimal dynamical models directly from time series data. In full analogy with cartography, we learn a representation of a system as an atlas of charts. This approach allows us to obtain dynamical models of the lowest possible dimension, leads to computational benefits, and can separate state space into regions of distinct behaviors. https://arxiv.org/abs/2108.05928
Event Details
Date/Time:
Abstract
Model reduction techniques have previously been applied to evolve the Navier-Stokes equations in time, however finding the minimal dimension needed to correctly capture the key dynamics is not a trivial task. To estimate this dimension we trained an undercomplete autoencoder on weakly chaotic vorticity data (32x32 grid) from Kolmogorov flow simulations, tracking the reconstruction error as a function of dimension. We also trained a discrete time stepper that evolves the reduced order model with a nonlinear dense neural network. The trajectory travels in the vicinity of relative periodic orbits (RPOs) followed by sporadic bursting events. At a dimension of five (as opposed to the full state dimension of 1024), power input-dissipation probability density function is well-approximated; Fourier coefficient evolution shows that the trajectory correctly captures the heteroclinic connections (bursts) between the different RPOs, and the prediction and true data track each other for approximately a Lyapunov time.
Event Details
Date/Time:
Event Details
Date/Time:
Title: Search for Southern Sky Neutrino Sources with IceCube
Abstract: The IceCube Neutrino Observatory has measured a flux of astrophysical neutrinos. More recently, it has used muon-neutrino datasets to present evidence for one source, a flaring blazar known as TXS 0506+056. However, the sources responsible for the majority of the astrophysical neutrino flux remain elusive. Galactic sources and the galactic plane have long been hypothesized as potential neutrino sources, however searches in the southern sky are limited due to the high background of down going muons produced in the atmosphere. In this work we present a new neutrino dataset relying heavily on Deep-Neural-Networks (DNN) to select cascade events produced from neutral-current interactions of all flavors and charged-current interactions with flavors other than muon-neutrino. The speed of DNN based selections allows the event selection to be performed in near-real time with a single GPU. Cascade events have reduced angular resolution when compared to muon-neutrino events, however the resulting dataset has a much lower energy threshold in the southern sky and a lower background rate. These benefits lead to an improved sensitivity to sources in the southern sky when compared to muon-neutrino datasets. This improvement is particularly promising for identifying galactic sources including the galactic plane itself.
Event Details
Date/Time:
Abstract
Quantum entanglement is a key driver of exotic physics in quantum materials, but it historically has been extremely hard to measure. In the first part of this talk I will discuss recent experiments showing how quantum spin entanglement can be extracted from neutron data using the tools of quantum information theory. We applied these protocols to 1D spin chains KCuF3, Cs2CoCl4, and triangular lattice KYbSe2. Of the protocols three we tested, we found Quantum Fisher Information (QFI) to be the most experimentally robust. Crucially, these protocols do not require theoretical models, which are only sometimes available for 2D and 3D quantum systems. In the second part of the talk, I discuss a separate set of experiments on the Heisenberg spin chain KCuF3 showing Kardar-Parisi-Zhang (KPZ) hydrodynamics at room temperature. By comparing with theoretical simulations, we show that the high temperature dynamic exponent of KCuF3 is inconsistent with ballistic or diffusive transport, indicating instead superdiffusive KPZ behavior. This verifies a recent theoretical prediction, and shows the influence of quantum effects even at room temperature
Event Details
Date/Time:
1. Speaker Kevin Zeng
Deep Reinforcement Learning Using Data-Driven Reduced-Order Models Discovers and Stabilizes Low Dissipation Equilibria
Deep reinforcement learning (RL), a data-driven method capable of discovering complex control strategies for high-dimensional systems, requires substantial interactions with the target system, making it costly when the system is computationally or experimentally expensive (e.g. flow control). We mitigate this challenge by combining dimension reduction via an autoencoder with a neural ODE framework to learn a low-dimensional dynamical model, which we substitute in place of the true system during RL training to efficiently estimate the control policy. We apply our method to data from the Kuramoto-Sivashinsky equation. With a goal of minimizing dissipation, we extract control policies from the model using RL and show that the model-based strategies perform well on the full dynamical system and highlight that the RL agent discovers and stabilizes a forced equilibrium solution, despite never having been given explicit information about this state’s existence.https://arxiv.org/abs/2104.05437
2. Speaker Carlos Perez de Jesus
Data-driven estimation of inertial manifold dimension for chaotic Kolmogorov flow and time evolution on the manifold
Model reduction techniques have previously been applied to evolve the Navier-Stokes equations in time, however finding the minimal dimension needed to correctly capture the key dynamics is not a trivial task. To estimate this dimension we trained an undercomplete autoencoder on weakly chaotic vorticity data (32x32 grid) from Kolmogorov flow simulations, tracking the reconstruction error as a function of dimension. We also trained a discrete time stepper that evolves the reduced order model with a nonlinear dense neural network. The trajectory travels in the vicinity of relative periodic orbits (RPOs) followed by sporadic bursting events. At a dimension of five (as opposed to the full state dimension of 1024), power input-dissipation probability density function is well-approximated; Fourier coefficient evolution shows that the trajectory correctly captures the heteroclinic connections (bursts) between the different RPOs, and the prediction and true data track each other for approximately a Lyapunov time.
Event Details
Date/Time:
Abstracts
1. Speaker Alec Linot: Modeling chaotic spatiotemporal dynamics with a minimal representation using Neural ODEs
Solutions to dissipative partial differential equations that exhibit chaotic dynamics often evolve to attractors that exist on finite-dimensional manifolds. We describe a data-driven reduced order modelling (ROM) method to find the coordinates on this manifold and find an ordinary differential equation (ODE) in these coordinates. The manifold coordinates are found by reducing the system dimension via an undercomplete autoencoder – a neural network that reduces then expands dimension – and an ODE is learned in this coordinate system with a Neural ODE. Learning an ODE, instead of a discrete time-map, allows us to evolve trajectories arbitrarily far forward, and allows for training on unevenly and/or widely spaced data in time. We test on the Kuramoto-Sivashinsky equation for domain sizes that exhibit spatiotemporally chaos, and find the ROM gives accurate short- and long-time statistics with training data separated up to 0.7 Lyapunov times.
https://arxiv.org/abs/2109.00060
2. Speaker Kevin Zeng: Deep Reinforcement Learning Using Data-Driven Reduced-Order Models Discovers and Stabilizes Low Dissipation Equilibria
Deep reinforcement learning (RL), a data-driven method capable of discovering complex control strategies for high-dimensional systems, requires substantial interactions with the target system, making it costly when the system is computationally or experimentally expensive (e.g. flow control). We mitigate this challenge by combining dimension reduction via an autoencoder with a neural ODE framework to learn a low-dimensional dynamical model, which we substitute in place of the true system during RL training to efficiently estimate the control policy. We apply our method to data from the Kuramoto-Sivashinsky equation. With a goal of minimizing dissipation, we extract control policies from the model using RL and show that the model-based strategies perform well on the full dynamical system and highlight that the RL agent discovers and stabilizes a forced equilibrium solution, despite never having been given explicit information about this state’s existence.
https://arxiv.org/abs/2104.05437
Event Details
Date/Time:
What are the mysterious sources of the most energetic particles ever observed? What astrophysical sources produce very energetic neutrinos? How do particles interact at extreme energies? Building on the progress achieved by the ground-based observations we are developing space and sub-orbital missions to answer these questions. The Extreme Universe Space Observatory (EUSO) on a super pressure balloon (SPB) is designed to detect the highest energy astroparticles from above. EUSO-SPB1 flew in 2017 with a fluorescence telescope. EUSO-SPB2 is being built to observe both fluorescence and Cherenkov from ultrahigh and very high energy cosmic rays and neutrinos. These sub-orbital missions pave the way to space missions such as POEMMA, the Probe Of Extreme Multi-Messenger Astrophysics, a space mission designed to discover the sources of UHECRs and to observe neutrinos above 20 PeV from energetic transient events. EUSO-SPB2 and POEMMA will open new multi-messenger windows onto the most energetic events in the Universe, enabling the study of new astrophysics and particle physics at these extreme energies.
Event Details
Date/Time:
Pages
